Grasping the Art of Overcoming Information Collection Limitations in Google Analytics for Better Decision-Making
In the world of digital analytics, the ability to remove meaningful insights from data is critical for informed decision-making. Google Analytics stands as an effective tool for organizations seeking to recognize customer habits, track conversions, and optimize their on-line presence. Information collection constraints within this system can hinder the accuracy and depth of the info collected. What Data Does Google Analytics Prohibit Collecting?. To really harness the potential of Google Analytics for calculated decision-making, grasping the art of overcoming these restrictions is important. By utilizing tactical approaches and advanced strategies, organizations can raise their data quality, unlock hidden understandings, and lead the way for even more enlightened and efficient choices.
Information Quality Assessment
Information quality evaluation includes reviewing numerous aspects such as precision, completeness, uniformity, and timeliness of the data. One vital element to consider is information accuracy, which refers to how well the information shows the true worths of the metrics being determined.
Efficiency of information is another critical aspect in assessing data high quality. It includes guaranteeing that all essential data points are collected and that there are no voids in the information. Insufficient information can skew evaluation outcomes and prevent the capacity to get an extensive sight of user habits or internet site efficiency. Uniformity checks are additionally important in information high quality evaluation to determine any type of inconsistencies or anomalies within the data set. Timeliness is equally important, as outdated information might no more be relevant for decision-making processes. By focusing on information top quality evaluation in Google Analytics, services can improve the integrity of their analytics reports and make more informed decisions based on exact understandings.
Advanced Monitoring Strategies
Making use of innovative monitoring strategies in Google Analytics can substantially boost the depth and granularity of information gathered for even more comprehensive analysis and understandings. One such technique is occasion tracking, which permits for the surveillance of certain interactions on a web site, like clicks on switches, downloads of documents, or video clip sights. By executing event monitoring, companies can obtain a much deeper understanding of customer habits and engagement with their on-line web content.
Furthermore, personalized dimensions and metrics offer a method to customize Google Analytics to certain business needs. Personalized measurements permit the production of brand-new information factors, such as customer roles or customer segments, while custom-made metrics enable the monitoring of special efficiency indications, like income per user or average order value.
In addition, the use of Google Tag Supervisor can enhance the implementation of monitoring codes and tags across a web site, making it simpler to handle and deploy innovative tracking setups. By taking advantage of these innovative monitoring methods, organizations can unlock important insights and maximize their online techniques for far better decision-making.
Custom Dimension Implementation
To improve the deepness of information accumulated in Google Analytics past sophisticated tracking techniques like occasion monitoring, services can apply custom-made dimensions for more tailored insights. Customized measurements allow companies to define and accumulate particular data points that relate to their one-of-a-kind goals and goals (What Data Does Google Analytics Prohibit Collecting?). By assigning custom measurements to various aspects on a site, such as user communications, demographics, or session details, organizations can this hyperlink get an extra granular understanding of just how individuals involve with their on the internet buildings
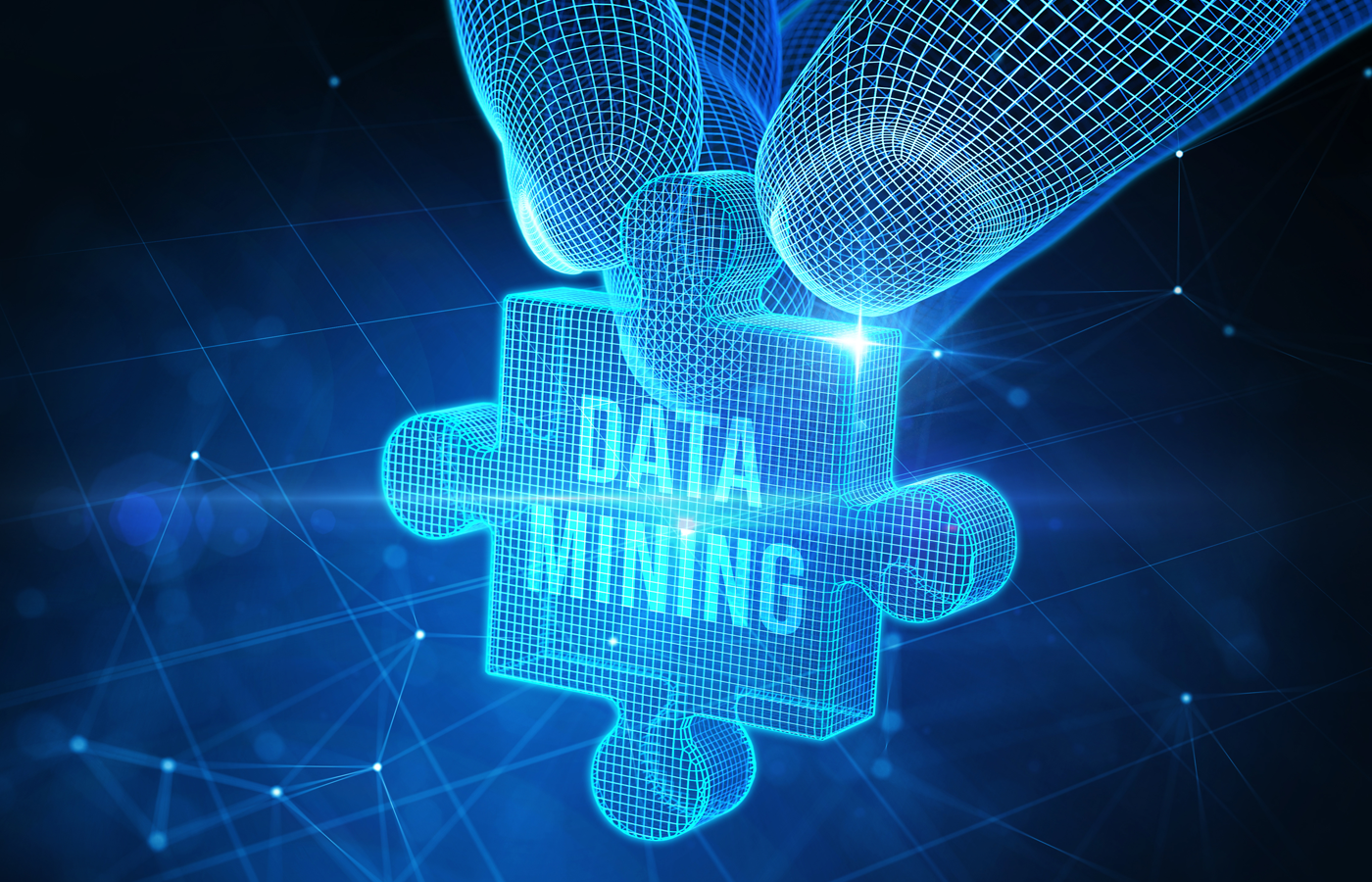
Acknowledgment Modeling Approaches
Efficient attribution modeling is crucial for comprehending the effect of different advertising and marketing channels on conversion paths. By employing the right acknowledgment model, organizations can accurately connect conversions to the suitable touchpoints along the consumer trip. One typical attribution design is the Last Communication model, which provides credit score for a conversion to the last touchpoint an individual connected with before converting. While this design is very easy and easy to implement, it typically oversimplifies the client journey, disregarding the impact of various other touchpoints that added to the conversion.
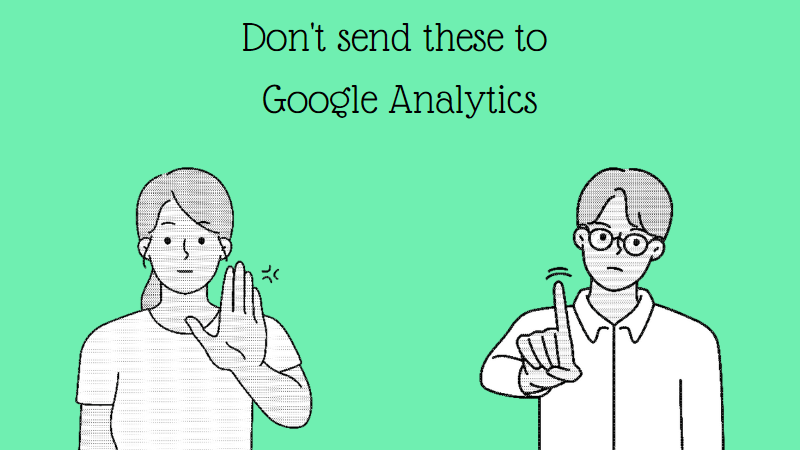
Information Tasting Avoidance
When handling big volumes of information in Google Analytics, overcoming data tasting is essential to ensure accurate understandings are obtained for educated decision-making. Information tasting occurs when Google Analytics approximates patterns in data instead of have a peek here evaluating the total dataset, potentially bring about skewed outcomes. To prevent information tasting, one effective technique is to decrease the date variety being assessed. By concentrating on much shorter period, the chance of encountering sampled information reductions, giving an extra exact representation of user actions. In addition, making use of Google Analytics 360, the premium variation of the system, can help minimize sampling as it permits greater information thresholds before tasting starts. Carrying out filters to limit the information being evaluated can also assist in staying clear of sampling concerns. By taking these positive actions to minimize information sampling, organizations can draw out more exact insights from Google Analytics, leading to far better decision-making and enhanced general efficiency.
Verdict
To conclude, grasping the art of getting over data collection restrictions in Google Analytics is crucial for making informed decisions. By carrying out a thorough data high quality evaluation, applying advanced tracking methods, making use of custom dimensions, employing attribution modeling methods, and staying clear of data sampling, companies can make sure that they have precise and reliable data to base their choices on. This will inevitably cause extra reliable approaches and far better end results for the company.
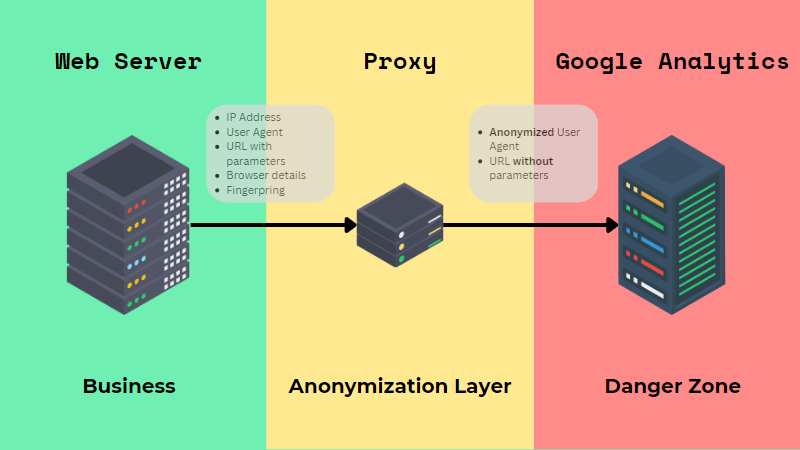